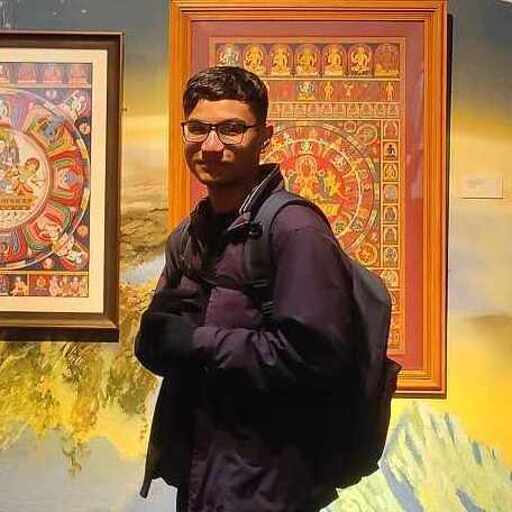
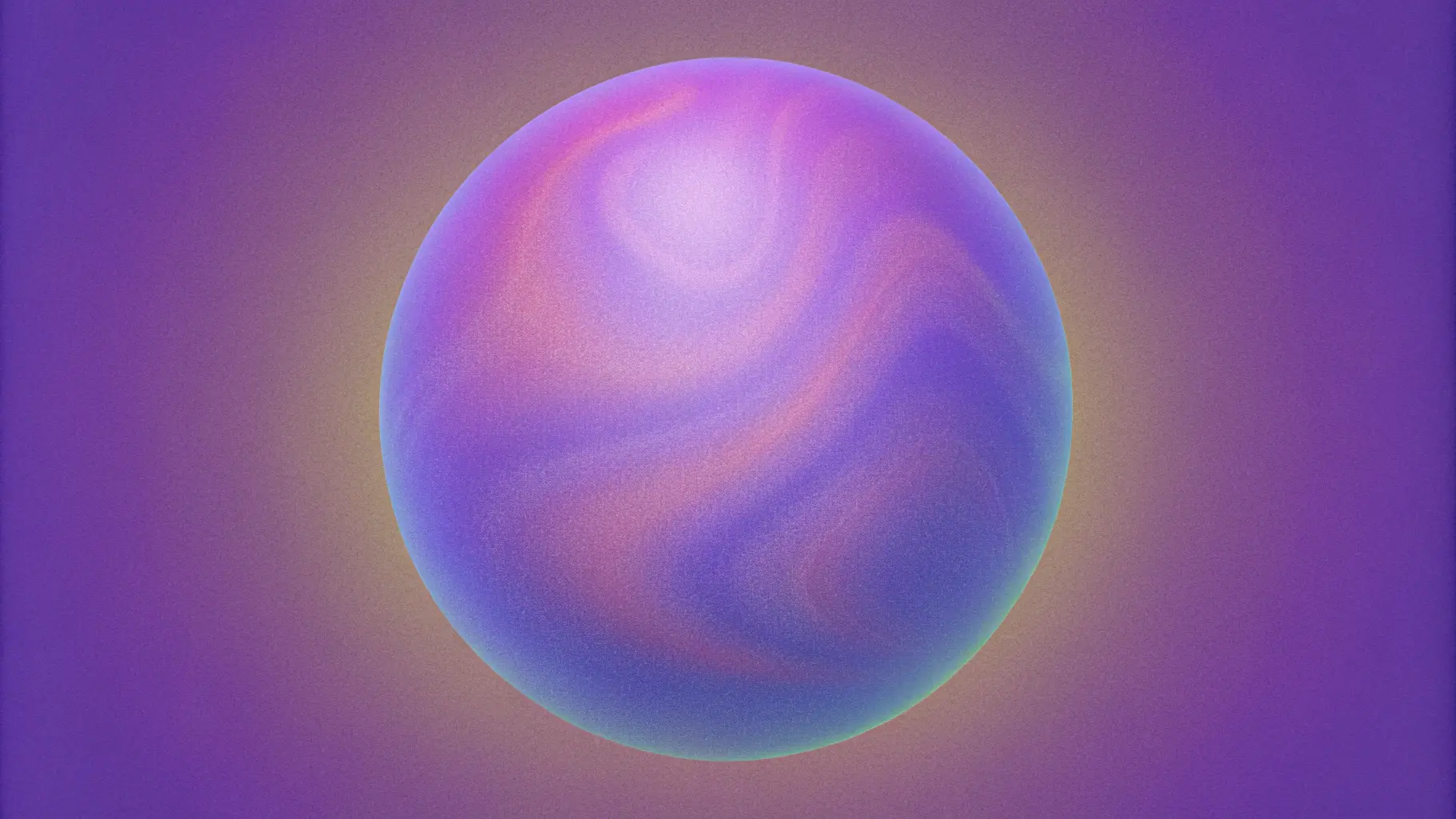
Face blurring is essential for protecting privacy in videos, especially for content creators, businesses, and organizations handling sensitive footage. This step-by-step guide demonstrates how to build an automated face blurring system using Sieve's AI API and Python. You'll learn to create a robust pipeline that can detect and blur faces in real-time while maintaining video quality.
Why Blur Faces in Videos?
Face blurring serves several critical purposes:
- Privacy Protection: Safeguards individual identities when consent isn't available
- Legal Compliance: Meets GDPR, CCPA, and other privacy regulations
- Security: Protects individuals in sensitive or vulnerable situations
- Content Moderation: Enables safe content sharing while maintaining anonymity
Key Features of Our Face Blurring Solution
This implementation effectively handles:
- Multiple faces in crowded scenes
- Various face angles and positions
- Dynamic camera movements
- Rapid scene transitions
- Real-time face detection and blurring
Building an Automated Face Blurring Pipeline
Let's go through each step of this efficient face blurring pipeline in detail alongside its corresponding code for better clarity and understanding.
Initial Setup
We use the Sieve API to build this pipeline. To get started with Sieve, sign up and get your API key, then install the Python client and log in.
pip install sievedata
sieve login
AI-Powered Face Detection
We'll use the sieve/yolov8 function to automatically identify all the faces in our video:
import sieve
# Initialize video file
video = sieve.File("your_video_path")
# Run face detection
yolov8 = sieve.function.get("sieve/yolov8")
face_detections = yolov8.push(
video,
classes='face',
confidence_threshold=0.05,
models="yolov8l-face"
)
coordinates = face_detections.result()
Convert Video into Frames
Next, we convert the entire video into a sequence of frames, with each frame being individually processable.
import cv2
def extract_frames(video_path):
cap = cv2.VideoCapture(video_path)
frames = []
while True:
ret, frame = cap.read()
if not ret:
break
frames.append(frame)
cap.release()
return frames
frames = extract_frames(video.path)
print(f"Extracted {len(frames)} frames")
Apply Dynamic Blur
We'll use the OpenCV package to dynamically apply an elliptical blur to each extracted frame based on the previously obtained face coordinates as region of interest.
def apply_elliptical_blur(frame, box, margin=20, blur_strength=151):
x1, y1, x2, y2 = box['x1'], box['y1'], box['x2'], box['y2']
# Calculate ellipse parameters
center = ((x1 + x2) // 2, (y1 + y2) // 2)
axes = ((x2 - x1) // 2 + margin, (y2 - y1) // 2 + margin)
# Create and apply mask
mask = cv2.cvtColor(frame, cv2.COLOR_BGR2GRAY)
mask[:] = 0
cv2.ellipse(mask, center, axes, 0, 0, 360, 255, -1)
# Apply blur
blurred = cv2.GaussianBlur(frame, (blur_strength, blur_strength), 0)
# Combine original and blurred regions
result = cv2.bitwise_and(frame, frame, mask=~mask) + \
cv2.bitwise_and(blurred, blurred, mask=mask)
return result
# Process all frames
for coord in coordinates:
frame_idx = coord['frame_number']
if frame_idx >= len(frames):
continue
for box in coord['boxes']:
frames[frame_idx] = apply_elliptical_blur(frames[frame_idx], box)
Reconstruct the Video
Now, we'll reconstruct the video from the processed frames. We'll also get the FPS of the original video and save the video without audio.
def get_fps(video_path):
cap = cv2.VideoCapture(video_path)
fps = cap.get(cv2.CAP_PROP_FPS)
cap.release()
return fps
def save_video(frames, output_path, fps):
height, width = frames[0].shape[:2]
writer = cv2.VideoWriter(
output_path,
cv2.VideoWriter_fourcc(*'mp4v'),
fps,
(width, height)
)
for frame in frames:
writer.write(frame)
writer.release()
# Save video without audio
temp_video = "temp.mp4"
fps = get_fps(video.path)
save_video(frames, temp_video, fps)
Audio Reattachment
import subprocess
def reattach_audio(temp_video, original_video, output_path):
command = [
"ffmpeg",
"-loglevel", "warning",
"-y",
"-i", temp_video,
"-i", original_video,
"-c:v", "libx264",
"-preset", "fast",
"-c:a", "aac",
"-map", "0:v:0",
"-map", "1:a:0",
output_path
]
try:
subprocess.run(command, check=True, capture_output=True, text=True)
print(f"Video saved to {output_path}")
except subprocess.CalledProcessError as e:
print("Error reattaching audio:", e.stderr)
# Create final video with audio
reattach_audio(temp_video, video.path, "output.mp4")
Example Outputs
Benefits of AI-Powered Face Blurring
- Automated Detection: Eliminates manual review workload
- Consistent Tracking: Accurately follows faces across angles, motions, and scenes
- Cost Efficiency: Minimizes production expenses through automation
- Rapid Processing: Processes videos faster than manual editing
Conclusion
This automated face blurring solution provides a powerful, scalable way to protect privacy in video content. By combining Sieve's AI capabilities with OpenCV, you can process videos efficiently while maintaining high accuracy in face detection and blurring. This approach is particularly valuable for content creators, businesses, and organizations that need to handle sensitive video content at scale.
Ready to implement face blurring in your project? Join our Discord community for support or contact us at contact@sievedata.com.